In-Depth
You Already Know (Mostly) How To Secure AI: Conversation with AI Safety Alliance Chair Caleb Sima
How to balance the jackrabbit pace of AI innovation with IT's directive to keep data secure? Get better at basic security hygiene, according to a new AI thinktank.
Already behemoths in the cloud, Microsoft, Amazon and Google are each currently jockeying for position in the breakneck generative AI race. In the area of AI safety, however, the three rivals -- along with marquee AI firms OpenAI and Anthropic -- have put on a united front.
The companies recently announced their membership in the AI Safety Initiative, a working group formed under the auspices of the Cloud Security Alliance (CSA). Besides the aforementioned AI market players, the group also includes academics and government agencies. As John K. Waters wrote in this Pure AI article, the CSA AI Safety Initiative is "focused on the development of practical safeguards for current generative AI technologies, while also preparing for the advancements of more powerful AI systems in the future."
Caleb Sima, chair of the AI Safety Initiative, recently gave an interview to Pure AI to discuss some of the group's most pressing concerns. The following was our discussion, edited for brevity and clarity.
Pure AI: So, why do we need this AI safety initiative and why now?
Sima: We're seeing a lot of regulations and safety guidelines about the ethics [of AI]. Obviously, we don't want it to take over the world. There's also a lot of focus on whether AI will take jobs. I think there's a lot of great direction around some of the dangers in AI from a global perspective around the world. There are a lot of people focused on doing that.
But where I think there's less focus and less definition that needs to be created is around practical ways that we can safely deploy AI in an enterprise for security teams and engineers and the enterprise itself. This is less about, "Will AI take our jobs?" or, "Will AI take over the world?" This is more about how we as cybersecurity teams practically use AI in the right way inside of our enterprises. And that is why we put this together.
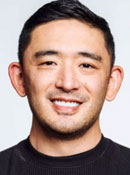
"Practically speaking, AI security is maybe 5 percent of what you do. Actually making AI safer is in the 95 percent that is the existing controls -- things that every enterprise and security team knows about already today."
Caleb Sima, Chair, Cloud Security Alliance AI Safety Initiative
I'll give you a great example. There was a lot of fear when AI first came out from security teams and enterprises around uploading private information into AI. This was the first reaction from security people: "Oh, you can't do that. We're just cutting off access." Actually, if you know and understand how the technology works and what it does, [you'd know that] it's not about blocking access. It's about treating this the same way we treat SaaS services today.
The AI itself is not where data leakage is the problem, right? It's not about trusting the LLM. It's about trusting the company that holds the LLM -- that is where the real issue comes in, and it's the education around that [that's needed] so that as CISOs and security practitioners, we understand what really is the problem and the practical guidelines that allow employees and customers to use LLMs properly without going into this fear.
What are the specific fears that you're hoping this working group will be able to address? Obviously, as you mentioned, security is a big one.
There are a lot of unknowns, right? AI as a space, both from a technology perspective and from a security perspective, is moving really fast. And I think the challenges that security teams and enterprises are having is understanding the technology, how it works. If you can understand the technology and how it works, you can also understand the risks that come with it. What we're trying to do is both educate security teams and enterprises around the technology...and also help them understand the risks that are associated with it.
For example, you've probably heard lots of things around "data poisoning," "prompt injection" and "data leakage" as these are huge security risks. But the the issue is, if you don't understand the technology, then how do you really know whether data leakage or data poisoning are real risks for you? That's a big question for enterprises. What we want to do is come to these enterprises and say, "Here's how the technology works. Here are the common ways of deploying it. Here are areas where data leakage is a problem for you. And here are areas where it's just not a problem for you."
In your security team, you may even have questions about, "How do I test whether data leakage is a problem for me? Does my pentesting team do that or does my engineering team do that?" We can provide guidance to say, "In your team, here's how you test for data leakage. Here are the situations where data leakage is a problem for you. Here are the teams and people responsible that would be best in place to test for data leakage. And, by the way, here are the educational materials they can learn so that they can understand how to do that properly."
It sounds like this new body will be able to work directly with companies in a sort of consultative way. Is that accurate? In what way will companies be able to access the findings, resources and research that this group will develop?
We're going to be producing a portal. A lot of the information that we're building is going to be available via the portal, from guidelines, documents, even to security teams. For example, I might be a detection engineer on a security team. How does my job change because of AI? You can go, "I'm a detection engineer. Here's where I'm at. What does AI do for me? What do I need to look for? How does my job change?" And it will help guide you to those practical things that you need to know in order to do the job right.
What is the group working on now? What's the most pressing problem?
I'll give you an example of what we're in the middle of right now that is going to be massively helpful. Many people, when they say, "AI security," they just say, "We just need to secure AI." The challenge is, practically speaking, "AI security" is maybe 5 percent of what you do. Actually making AI safer is in the 95 percent that is the existing controls -- things that every enterprise and security team knows about already today.
Let me give you an example. A model is just a file. If you store that file in an [Amazon Web Services] S3 bucket or in some data store, the permissions and the configurations that put the right security controls around protecting that model is the same stuff that you've dealt with. It's not magic. It's the same thing that you deal with every day. However, if someone gets access to that file, how you determine whether that model has the right integrity or not may be a very AI-specific problem that we need to figure out. But what we do know is 95 percent of protecting AI is about basic infrastructure application security controls that people are very used to.
So right now, what the group is in the middle of is defining...what is really AI-specific versus what's all the rest of it -- the standard controls that you very much know how to do. And I think defining that line is really good.
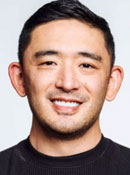
"My bet -- and I will almost guarantee this -- is that models and AI will end up making decisions and will end up creating actions. And how you monitor and manage that is going to be an interesting challenge."
Caleb Sima, Chair, Cloud Security Alliance AI Safety Initiative
As you mentioned, the AI space is moving incredibly fast right now. How do you foresee the AI Safety Initiative evolving in the next couple of months as AI evolves? Are there any developments you foresee having to prepare for, and that you then have to warn companies to prepare for, too?
If you look at LLMs today, they're mostly used as search oracles or for [writing-generation]. Organizations are trying to figure out how to use LLMs to summarize information and communicate with different stakeholders. But when we think about the future and how things may change in two or three months, you start realizing that LLMs are evolving. It's not just the fact that we're communicating with it with text. We're communicating with it with voice, with pictures and photos, and then in the next six months, we're going to be communicating with it with video.
I also think that the roles of LLMs and generative AI in enterprises will start changing. As I said, [right now] it's a lot about searching or generating or creating. But when LLMs start acting and deciding on things, when they start making decisions inside of an organization [and] they start having access to things and being able to execute things -- which is coming down the road -- the security challenge becomes much more complex. A great example is...the AI personal assistant. A personal assistant has to have access to my email and my social media. Well, how do I prevent AI from posting my email to my social media? It's obvious to any [executive assistant] not to do that, but for an LLM, how does that decision get made and how do you ensure that decision doesn't happen?
So I think the security challenges become very, very interesting as we move down the road. From our perspective at CSA, not only do we have to think about the structure of the problems we're practically dealing with today, but also when LLMs change their roles, what does that mean for security teams and enterprises? And what types of problems do we have to start solving for?
I think as you look forward in the future, my bet -- and I will almost guarantee this -- is that models and AI will end up making decisions and will end up creating actions. And how you monitor and manage that is going to be an interesting challenge.
What AI development are you personally excited to see in the next couple of months?
What I would say most excites me is seeing which LLMs can start really learning and understanding real-time data. That, I think, is something that is both very exciting and very challenging. Today, LLMs have a very specific context window that's somewhat limited in its ability to make decisions, and it's trained on a set of data that is a one-time snapshot. But when we start thinking about LLMs, say, making decisions, it's going to make decisions off of real-time data. So, either the context window in LLMs is going to get much, much larger -- potentially infinite -- or the fine-tuning processes of LLMs are going to get fast enough and short enough that you can effectively start fine-tuning models on the fly.