In-Depth
Anthropic's Vision for a Third-Party AI Testing 'Regime': Top 6 Takeaways
AI safety testing is a team sport, says the maker of the Claude AI models and chatbot, and "the best way to avoid societal harm" caused by AI.
The biggest players in the generative AI space all publicly agree that AI misuse is inevitable, pernicious and their responsibility to fight; some follow rigorous, self-imposed protocols to mitigate that misuse, if only internally. Governments around the world also recognize the threat of AI misuse, particularly to national security and election integrity, and have taken up arms against would-be bad actors in the form of regulations and guidelines.
These approaches, however, have so far been uncoordinated, slap-dash and limited.
Anthropic, maker of the Claude AI model and a self-described "AI safety" company, this week described its vision of "an effective ecosystem for third-party testing and evaluation of AI systems," one that's supported by governments, academic institutions and private companies alike, all united in the goal of creating trustworthy AI technologies and methodically weeding out their potential for abuse, while still maintaining an open and competitive AI marketplace.
At this point, such an ecosystem still seems like a distant milestone -- but, Anthropic argued, that distance is the point.
"Because broadly commercialized, general purpose AI is a relatively new technology, we don't think the structure of this ecosystem is clear today and it will become clearer through all the actors above running different testing experiments," the company wrote in a blog post Monday. "We need to start working on this testing regime today, because it will take a long time to build."
Anthropic's blog, titled "Third-party testing as a key ingredient of AI policy," is worth reading in full, but here are the most notable points.
1. The Time Is Now...But Also Later
With over 50 countries holding elections this year, state-sponsored cyberattacks on the rise and multiple international conflicts taking place at the same time, there is a clear and immediate need for standardized AI safety regulations now.
"Today's frontier AI systems demand a third-party oversight and testing regime to validate their safety," Anthropic said. "In particular, we need this oversight for understanding and analyzing model behavior relating to issues like election integrity, harmful discrimination, and the potential for national security misuse."
However, because of the rapid pace of AI innovation, any regulation must be treated as a living document, one that's subject to regular audits and systematic revisions. Essentially, Anthropic suggests, it must be a process of trial and error spread over a number of years.
"[W]e think there's a chance that today's approaches to AI development could yield systems of immense capability, and we expect that increasingly powerful systems will need more expansive testing procedures," Anthropic said, adding, "[D]esigning this regime and figuring out exactly what standards AI systems should be assessed against is something we'll need to iterate on in the coming years -- it's not obvious what would be appropriate or effective today, and the way to learn that is to prototype such a regime and generate evidence about it."
2. The Ingredients of an 'Effective Third-Party Testing Regime'
Effective AI regulations must have these four objectives, per Anthropic:
- Engender widespread trust in AI.
- Not be prohibitively difficult for smaller, less-resourced companies to clear.
- Only apply to "computationally-intensive, large-scale systems" (i.e., a vanishingly small proportion of all AI systems).
- Enable cooperation between countries via "shared standards."
The company also identifies two integral ingredients to get there -- the most basic building blocks of a solid AI regulatory system:
- Tried and true tests for measuring an AI system's potential for misuse.
- Trusted third parties to administer those tests.
So, what does such a testing framework actually look like? Anthropic lists six requirements:
- A "shared understanding" between all AI stakeholders of their goals.
- A trial period in which companies do mock runs of the AI tests to make sure they're sustainable.
- A two-part testing process, the first part being an automated test that's "biased towards avoiding false negatives," and the second being a human-led test looking specifically at problems identified in Part 1.
- Funding for the agencies that will be tasked with validating these tests.
- "A carefully scoped set of mandated tests - we'll need specific, legally mandated tests where it becomes clear there are poor incentives for industry self-governance, and the benefits of public safety from government oversight outweigh the regulatory burdens."
- Proof that the tests are reliable and useful, but not prohibitively difficult to administer.
3. Preempting Regulators' Worst Instincts
Regulation is an imperfect science. Anthropic identifies potential regulatory pitfalls in its blog, drawing on past history to anticipate how and why AI regulations might fail. At one point, for instance, it anticipates reactionary policies that are both draconian and useless:
In addition to obviously wanting to prevent major [AI-caused] accidents or misuse for its own sake, major incidents are likely to lead to extreme, knee-jerk regulatory actions, leading to a "worst of both worlds" where regulation is both stifling and ineffective. We believe it is better for multiple reasons to proactively design effective and carefully thought through regulation.
At another, it warns against regulations that, in a bid to ensure the highest level of product safety, unintentionally stifle competition:
The stakes are high: if we land on an approach that doesn't accurately measure safety but is easy to administer, we risk not doing anything substantive or helpful. If we land on an approach that accurately measures safety but is hard to administer, we risk creating a testing ecosystem that favors companies with greater resources and thus reduces the ability for smaller actors to participate.
It suggests that regulations can't be too rigorous, or else they risk being considered by enforcers to be more trouble than they're worth:
When developing our policy positions, we assume that regulations tend to create an administrative burden both for the party that enforces the regulation (e.g, the government), and for the party targeted by the regulation (e.g, AI developers). Therefore, we should advocate for policies that are both practical to enforce and feasible to comply with.
They also can't be too comprehensive, or else they might warp future corrective efforts:
We also note that regulations tend to be accretive -- once passed, regulations are hard to remove. Therefore, we advocate for what we see as the "minimal viable policy" for creating a good AI ecosystem, and we will be open to feedback.
4. Beware 'Regulatory Capture'
Perhaps the biggest policy minefield to avoid, in Anthropic's opinion, is "regulatory capture," which describes a situation in which regulators favor the interests of a few individual stakeholders over the interests of consumers at large.
Anthropic warns against creating a less-than-open AI market a few times in its blog, but nowhere as explicitly as it does in a section titled "Aspects of AI policy we believe are important to discuss." In fact, there are only two "aspects" discussed in this section, and regulatory capture is one of them (see No. 6 for the other).
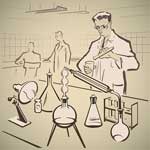
"We've come to believe that testing is fundamental to the safety of our systems -- it's not only how we better understand the capabilities and safety properties of our own models, but also how third-parties can validate claims we make about AI systems."
Anthropic, "Third-party testing as a key ingredient of AI policy," March 2024
"It's important that the AI ecosystem remains robust and competitive," Anthropic says, at the same time conceding that "[a]ny form of policy can suffer regulatory capture by a sufficiently motivated and well-resourced actor: for example, a well-capitalized AI company." (Anthropic doesn't name names, but "well capitalized" can refer to multiple companies at this point, from OpenAI to Mistral to Anthropic itself.)
A testing framework that hands the reins to "a diverse ecosystem of different organizations" can avoid giving outsized power to a small handful of large organizations, argues Anthropic: "We think that focusing on the development of third-party testing capacity can reduce the risk of regulatory capture and create a level playing field for developers."
5. National Security Is Ripe for AI Testing
"When it comes to tests, we can already identify one area today where testing by third-parties seems helpful and draws on the natural strengths of governments: national security risks," Anthropic says. "We should identify a set of AI capabilities that, if misused, could compromise national security, then test our systems for these capabilities. Such capabilities might include the ability to meaningfully speed up the creation of bioweapons or to carry out complex cyberattacks."
Anthropic suggests that governments should not delay in setting up robust AI testing programs now to address the near and present danger of AI-driven cybersecurity attacks. Given that governments frequently trade in sensitive data, "government agencies may carry out the tests directly," says Anthropic.
Overall, Anthropic's post underscores the role of government in fostering a healthy AI ecosystem more than it does the roles of universities and the private sector. It follows that many of the industry changes it advocates for are related to government. For instance, it is:
- Committing to helping the U.S. government develop ways to test AI systems for potential problems related to national security.
- Urging governments to create and support infrastructure focused on AI research.
- Urging more funding for government AI testing in general. "In the US," Anthropic says, "we specifically advocate for greater funding for [the National Institute of Standards and Technology]."
- Advocating for governments to create their own networks that researchers can tap for AI-capable compute power -- essentially, their own versions of the National Artificial Intelligence Research Resource in the United States.
6. The Open Source AI Model Problem
Besides regulatory capture (No. 4 in this article), the other pressing policy concern that Anthropic highlighted centers around open source, or openly disseminated, AI models -- specifically, their potential to become vehicles for AI misuse. Openness is critical to technological advancements, Anthropic concedes, including the ones happening in AI today. However, it tends to be at cross-purposes with the goal of -- in Anthropic's words -- "societal safety."
"We believe that the vast majority of AI systems today (perhaps even all of them) are safe to openly disseminate and will be safe to broadly disseminate in the future," it said, while adding, "If -- and 'if' is a key and unresolved point -- increasingly capable AI models can lead to detrimental effects, or hold the possibility of catastrophic accidents, then we'll need to adjust the norms of what is openly disseminated at the frontier."
Anthropic suggests two broad changes to harden open source AI systems, both of which it expects will be "inherently very costly" but nevertheless "necessary":
- "[E]nsure that AI developers release their systems in a way that provides strong guarantees for safety," for example by creating ways to identify and block efforts to abuse their systems, or by limiting how much the open source community can fine-tune models.
- "[E]xperiment with disclosure processes, similar to how the security community has developed norms around pre-notification of disclosures of zero days."
Notably, Anthropic says that as a for-profit company, it shouldn't be the final arbiter of how open source companies police their systems ("Anthropic is not an impartial actor here," reads the blog, emphasis Anthropic's). That's a role for trusted third-party testers.
"[T]o resolve questions of open source models," says Anthropic, "we need legitimate third parties to develop testing and evaluation approaches that are broadly accepted as legitimate, [and] we need these third parties (or other trusted entities) to define a narrow and serious set of misuses of AI systems as well as adverse AI system behaviors."